Driving Enterprise Success with AI-Enhanced Information Management
- Padmanaban D
- Apr 2
- 9 min read
Artificial Intelligence (AI) has evolved remarkably over the decades, transforming from theoretical concepts to a driving force behind modern technological advancements. Its journey began in the 1950s when Alan Turing introduced the idea of machine intelligence with the Turing Test, and John McCarthy coined the term "Artificial Intelligence." The 1970s saw the rise of expert systems like MYCIN, which applied rule-based logic to problem-solving. By the 1990s, machine learning and data mining gained prominence, culminating in IBM’s Deep Blue defeating world chess champion Garry Kasparov in 1997. The 2010s marked the deep learning revolution, with AI systems like Google’s AlphaGo surpassing human intelligence in complex games. Today, AI is an integral part of daily life, powering virtual assistants, autonomous vehicles, and advanced healthcare diagnostics, while sparking critical discussions on ethics and regulations. This historical progression underscores AI’s transformative impact, setting the stage for its role in enterprise success, particularly in AI-enhanced information management.

The evolution of cloud computing through Infrastructure as a Service (IaaS), Platform as a Service (PaaS), and Software as a Service (SaaS) has transformed how enterprises manage their IT resources, paving the way for AI to revolutionize information management. IaaS provides the foundational layer, offering virtualized servers, storage, and networking, which businesses traditionally managed on-premises. PaaS builds on this by adding runtime environments, middleware, and operating systems, enabling developers to focus on building applications without worrying about underlying infrastructure. SaaS takes it further, delivering fully managed applications and data directly to users, streamlining operations. As these cloud models matured, AI began to integrate seamlessly across these layers, enhancing data processing, automating workflows, and providing intelligent insights. This synergy between cloud services and AI has empowered enterprises to optimize information management, driving efficiency, innovation, and success in an increasingly data-driven world.
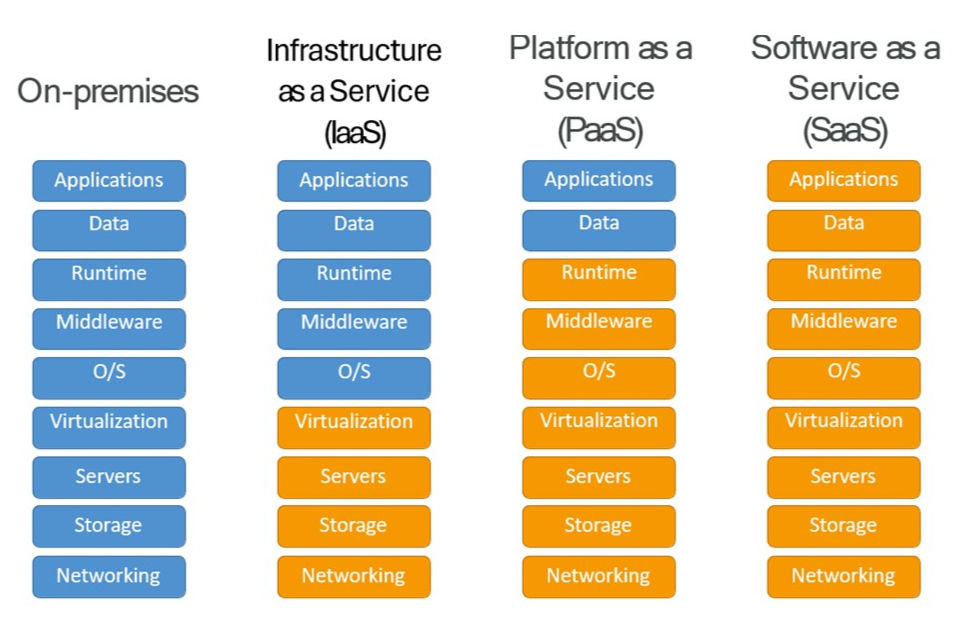
As I delve into AI trends based on discussions with global telecom, government, and utility providers, it is clear that enterprises are increasingly seeking AI-driven solutions to efficiently manage vast information repositories. In the context of the architecture I have analysed, I will highlight how AI is transforming knowledge retrieval, optimizing service delivery, and enhancing decision-making across these sectors.
Unlike traditional static databases, AI-powered knowledge bases offer dynamic capabilities to aggregate, structure, and retrieve information in real time. These systems seamlessly integrate data from diverse sources, including enterprise applications, customer interactions, and external data streams. By continuously learning and adapting, they provide contextually relevant responses, improving both operational efficiency and user experience. This evolving trend reflects a broader shift towards AI technologies that can rapidly process, analyze, and utilize information, driving smarter and more responsive business operations across industries.
Impact of AI in Enterprise
Through my engagements with global enterprises, I have observed how AI-driven knowledge bases analyse historical customer interactions, detect patterns in queries, and automate responses across digital channels such as chatbots, websites, and interactive voice response (IVR) systems. In the telecom industry, for example, AI systems provide real-time resolution to customer issues, reducing reliance on human agents for repetitive tasks.
The integration of AI into enterprise systems is vividly illustrated through a dynamic process that enhances decision-making and operational efficiency. AI captures data from diverse sources such as existing resolutions, pre-integrated modules, and other integrations, alongside user-generated inputs like logs, issues, and feedback. This data undergoes a sophisticated process where AI learns, understands, and extracts meaningful insights through topic recognition, data extraction, validation, and analysis of behavioural patterns. The result is a powerful output of contextual insights and suggested solutions, enabling enterprises to address challenges proactively, optimize workflows, and deliver personalized user experiences. By leveraging AI in this way, businesses can transform raw data into actionable intelligence, driving innovation and success in a competitive landscape.
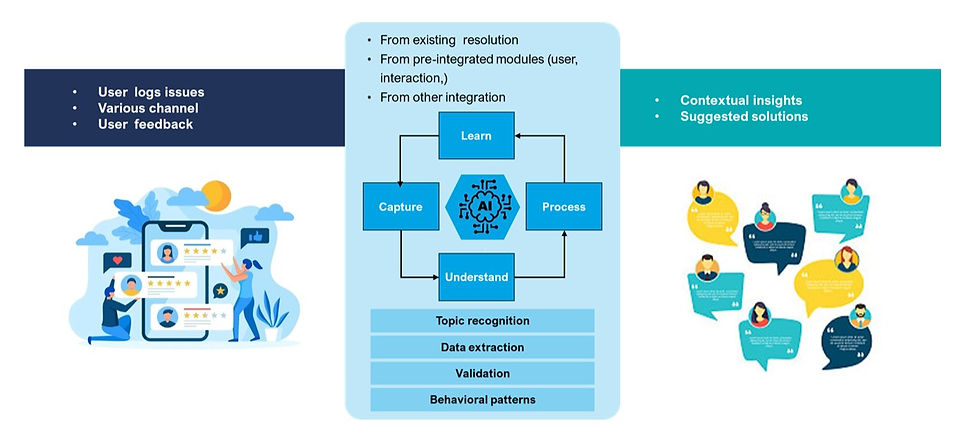
Similarly, in the utilities sector, AI-powered knowledge repositories provide real-time outage updates, analyse energy consumption trends, and recommend personalized cost-saving strategies. In government agencies, AI-driven self-service portals help citizens access public records, policy documents, and tax-related information, thereby enhancing operational efficiency and public engagement.
AI Integration with Enterprise Applications
A crucial aspect of AI-driven knowledge bases is their ability to seamlessly integrate with enterprise applications such as Customer Relationship Management (CRM), Enterprise Resource Planning (ERP), and IT Service Management (ITSM) systems. Through my research and discussions, I have seen enterprises benefit from AI-driven insights by pulling structured and unstructured data from these systems and processing it with Large Language Models (LLMs).
The architecture demonstrates a sophisticated framework where AI acts as the central intelligence layer, seamlessly bridging diverse enterprise systems to optimize operations and deliver significant business benefits. The AI integrates with communication and collaboration platforms like Microsoft Teams, WhatsApp, Slack, X, LinkedIn, and IVR systems, enabling it to ingest real-time data from key enterprise functions. These include customer service management for handling client inquiries, helpdesk and interaction systems for managing technical support, contract management for overseeing agreements, and invoice and payment management for tracking financial transactions. By leveraging custom memory, conversation history, and segment profile aggregation, the AI processes this data to retain context, maintain interaction continuity, and categorize users based on behavior and preferences, ensuring a deep understanding of enterprise dynamics. On the output side, the AI interacts with external applications via APIs, managing files, websites, and plain text, which ensures compatibility with a wide range of tools. The "Process Output/Retrieving Knowledge Based on the Input" mechanism allows the AI to deliver actionable outcomes, such as automated responses, detailed reports, or decision-making insights, while maintaining a feedback loop to continuously improve its performance.
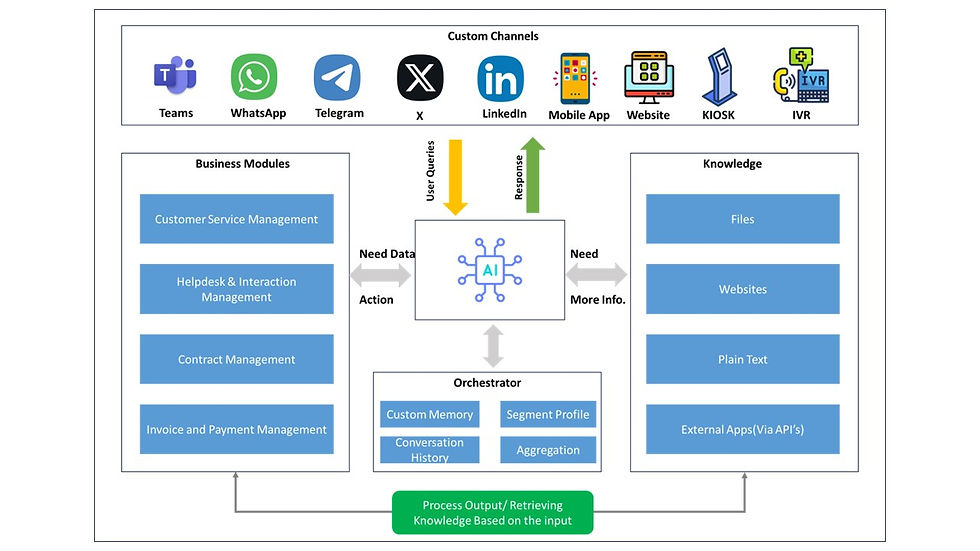
This integration yields substantial business benefits, including enhanced operational efficiency through automation of repetitive tasks like invoice processing or customer query resolution, reducing manual effort and errors. It improves customer satisfaction by enabling personalized, context-aware interactions, such as tailored support responses based on conversation history. The AI-driven insights from segment profiling and data aggregation empower enterprises to make informed decisions, optimize resource allocation, and identify new opportunities for growth. Additionally, the architecture breaks down silos between departments, fostering cross-functional collaboration and ensuring a unified view of operations. By streamlining workflows, reducing response times, and enhancing scalability through API-driven external integrations, businesses can achieve cost savings, improve agility, and maintain a competitive edge in a rapidly evolving market. Ultimately, this AI integration transforms enterprise applications into a cohesive, intelligent ecosystem that drives productivity, innovation, and long-term success.
Sample Use Cases per industry and Integrations
Industry | CRM Integration | ERP Integration | ITSM Integration |
Telecom | - AI-driven chatbots assist in handling customer queries about service disruptions, offering self-service options for troubleshooting. | - AI-powered CRM systems can analyze customer complaints and service requests, automating issue categorization, prioritization, and ticket routing to the correct department, improving operational efficiency. | - AI-powered ITSM systems in telecom help automate the management of service tickets by automatically categorizing and routing incidents based on severity. |
- AI links customer profiles to personalize interactions, offering tailored promotions or discounts based on usage patterns. | - Integration of AI with billing and payment systems, enabling AI to predict payment issues and offer solutions proactively. | - AI can predict service outages or network issues and proactively create tickets for resolution, improving response times and customer satisfaction. | |
- Automated follow-ups for unresolved complaints to ensure timely resolution. | - Automated response generation for common service-related issues, reducing wait times for customers. | ||
Utilities | - AI-driven CRM systems provide proactive service notifications to customers, such as alerts on maintenance schedules or service interruptions, based on real-time interaction data. | - AI integrated with ERP systems analyzes energy consumption trends. When customers inquire about high energy bills, the AI retrieves historical data, identifies anomalies, and recommends optimal usage patterns. | - AI-powered ITSM systems handle customer support requests related to outages or service issues, automatically providing status updates and estimated resolution times based on historical data. |
- Personalized recommendations for energy-saving practices based on customer usage patterns, improving customer engagement. | - AI helps optimize the energy grid by forecasting demand and managing resource allocation during peak times. | - AI prioritizes incoming service requests by severity, ensuring critical issues are addressed first. | |
- AI-based predictive maintenance helps detect potential system failures before they impact customers. | |||
Government | - AI-enhanced CRM systems in government agencies track constituent concerns and complaints, automating case management and prioritizing issues based on urgency or importance. | - AI integrated with ERP systems in government helps track and manage public resource allocation, such as water or electricity distribution, and optimizes supply chain logistics during crises. | - AI-enhanced ITSM systems in government agencies process citizen queries about property tax assessments, fetch relevant records, validate past transactions, and provide resolution pathways, reducing processing time and manual workload. |
- AI-driven systems help route citizen inquiries to the appropriate department, reducing delays and improving response times. | - AI-driven systems automate procurement processes, reducing delays and ensuring compliance with regulations. | - AI systems help track and manage IT infrastructure issues, ensuring government services remain operational with minimal downtime. | |
- AI automates ticket management for civil infrastructure maintenance, optimizing scheduling and resource allocation. |
Challenges Vs Best practices
Category | Challenges in AI-driven Knowledge Management | Best Practices for Implementing AI in Knowledge Bases |
Data Accuracy | Ensuring AI-generated responses remain precise and trustworthy. | Structured Data Modeling: Ensuring AI can efficiently process and retrieve information from enterprise applications. |
Bias Mitigation | Preventing biases in AI models, particularly in government applications where fairness is critical. | Hybrid Approach: Combining rule-based logic with AI-driven learning for optimal performance. |
Privacy & Security | Managing sensitive customer and public data while ensuring compliance with industry regulations. | Continuous Training: Regularly updating AI models with fresh data to enhance accuracy. |
Handling Complex Queries | Some ambiguous or intricate cases still require human oversight. | Human-in-the-Loop Monitoring: Implementing a review mechanism for high-impact AI-generated responses. |
Approach to Challenges | Organizations are addressing these challenges by deploying robust data validation mechanisms, continuous model training, and regulatory compliance frameworks. In discussions with government IT teams, some agencies have implemented AI audit systems that flag potentially biased responses for human review before finalizing replies to citizen queries. | Real-World Example: In utilities companies, a feedback loop has been established where human agents validate AI-generated energy-saving recommendations, refining the system’s accuracy over time |
Way of addressing the Enterprise Reservations in Adopting AI
Challenge | Description | Potential Solution |
Resistance to Change | Employees and leadership may be hesitant to adopt AI technologies due to fears of job displacement or disruption of established processes. | Foster a culture of innovation, offer training, and demonstrate AI’s potential benefits through pilot programs and gradual implementation. |
Lack of Clear Use Cases | Uncertainty around how AI can be practically applied to specific business needs or challenges, leading to hesitation in adoption. | Identify and define clear use cases that align with organizational goals, starting with high-impact, low-risk areas to demonstrate AI’s value. |
Legacy Systems and Integration Issues | Existing legacy systems may not be easily compatible with AI technologies, creating integration hurdles. | Focus on modular AI solutions that can integrate with legacy systems, and consider cloud-based solutions that facilitate easier compatibility. |
Data Challenges | Enterprises may face issues related to data quality, availability, or accessibility, which is critical for effective AI training and operation. | Ensure robust data management practices, invest in data cleaning and integration tools, and create centralized data repositories. |
Limited Understanding & Expertise | A lack of in-house AI knowledge and expertise can prevent enterprises from effectively implementing and managing AI-driven solutions. | Invest in training programs, hire or consult AI experts, and partner with AI service providers to build the necessary expertise internally. |
Implementation Costs | The initial investment required for AI development, infrastructure, and resources can be a significant barrier for enterprises. | Start with pilot projects to minimize risk, leverage cloud-based AI solutions for cost efficiency, and gradually scale up the AI adoption process. |
Integration Complexity | Aligning AI with existing enterprise systems like CRM and ERP can be complex and time-consuming. | Focus on a phased integration approach, ensuring AI solutions complement existing systems without disrupting operations. |
Regulatory Compliance | AI systems must adhere to industry regulations and data privacy laws, which may vary across regions and sectors. | Implement robust governance models, continuously monitor compliance, and consult with legal teams to ensure adherence to regulations. |
Standard LLM Models and Cost Considerations
LLM Model | Cost | Pricing Model | Key Considerations |
OpenAI GPT-4 | $0.03 - $0.12 per 1,000 tokens | Pay-per-use, depending on input and output token usage | Suitable for high-scale applications with fluctuating query volume |
Google Gemini AI | - Gemini 1.5 Flash: $0.00001875 - $0.000075 per 1,000 characters | Pay-per-use, based on input and output modalities and volume | Flexible pricing depending on specific enterprise requirements; detailed pricing on Google Cloud's Vertex AI page |
- Gemini 1.5 Pro: $0.0003125 - $0.00125 per 1,000 characters | |||
- Gemini 1.0 Pro: $0.000125 - $0.000375 per 1,000 characters | |||
Anthropic Claude AI | $0.08 - $0.12 per 1,000 tokens | Pay-per-use based on tokens processed | Competitive pricing with a focus on conversational AI applications |
Meta Llama | Free (open-source) | Requires self-hosting infrastructure | Suitable for organizations with technical resources for self-hosting |
Grok AI | - X Premium: $8/month | Subscription-based for individuals; Pay-per-use for enterprise API | Developed with specific integrations in mind; enterprise API solutions use token-based pricing |
- X Premium+: $16/month | |||
- Enterprise API: Input tokens at ~$2 per million, Output tokens at ~$15 per million | |||
AWS Q | - AWS Q Developer: $19/user/month | Subscription-based for users; Index pricing for document processing | Scalable AI services within the AWS ecosystem; additional charges for processing images, audio, and video |
- AWS Q Business Lite: $3/user/month | |||
- AWS Q Business Pro: $20/user/month | |||
- Index Pricing: $0.140 - $0.264 per hour per unit | |||
IBM Watsonx.ai | - Trial: Free | Subscription-based; pay-per-use for token processing | Enterprise-ready AI services with options for open-source and IBM foundation models |
- Standard: $1,050/month | |||
- IBM Models: $0.20 per 1M tokens | |||
-Third-Party Models: ~$20.85 per 1M tokens |
So what next ?
As AI continues to evolve, knowledge bases will expand to support multimodal inputs—text, voice, and visual data. For instance, telecom providers are exploring AI-powered video guides for troubleshooting network issues. Utilities companies are using AI-powered voice assistants to deliver real-time energy insights. Government agencies are likely to adopt AI-driven virtual assistants to guide citizens through complex bureaucratic processes using augmented and virtual reality (AR/VR).
From my discussions with industry experts and analysis of AI-driven knowledge base projects, it is evident that AI is fundamentally reshaping how industries interact with their customers and stakeholders. Organizations looking to implement AI-powered knowledge bases must embrace continuous innovation, integrate with enterprise applications, and adopt best practices to ensure optimal performance. The future of knowledge management is AI-empowered, unlocking new levels of automation, intelligence, and user engagement.
This was insightful! Given the rapid advancements in AI, which breakthrough apart from ChatGPT, Tesla Autopilot, and AlphaGo, do you find most exciting? Which AI technology do you think will be in high demand soon?